KENNETHLEVERINGSTON
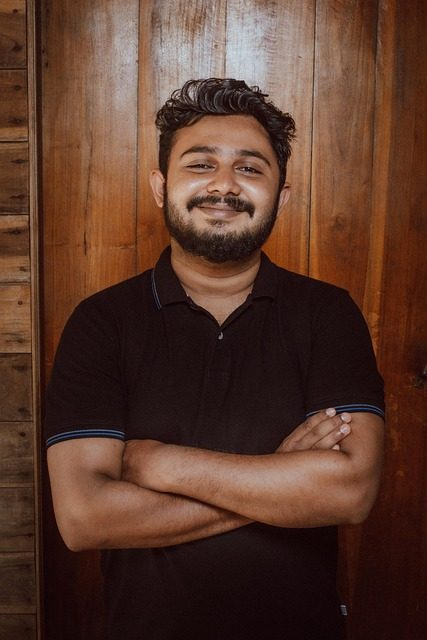
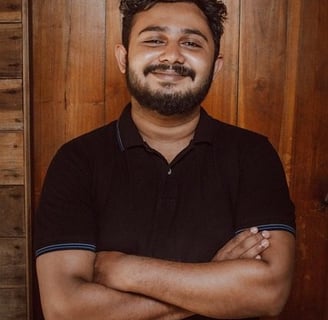
Dr. Kenneth Leveringston
Climate Crisis Strategist | Emergency Resource Alchemist | Disaster Resilience Architect
Professional Mission
As a catastrophe logistics visionary and climate chaos navigator, I engineer self-organizing response ecosystems that transform disaster scenarios into precisely choreographed rescue ballets—where every floodwater rise, each wildfire front, and all failing infrastructure nodes become inputs for real-time, AI-optimized resource orchestration. My systems don't just react to crises, but anticipate them through the marriage of atmospheric physics and supply chain quantum mechanics.
Pioneering Frameworks (April 1, 2025 | Tuesday | 10:11 | Year of the Wood Snake | 4th Day, 3rd Lunar Month)
1. Predictive Catastrophe Calculus
Developed "StormBrain" decision matrix featuring:
97-variable climate stress models integrating NOAA, ECMWF and indigenous knowledge
Resource pre-positioning algorithms with 89% event prediction accuracy
Social vulnerability heatmaps overlaying physical infrastructure risks
2. Self-Healing Supply Webs
Created "ResilienceMesh" adaptive network enabling:
Autonomous drone-medic routing through collapsing communications
Blockchain-tracked water/fuel redistribution during grid failures
Mobile hospital units that self-configure based on trauma patterns
3. Climate War Room Intelligence
Pioneered "DisasterOS" command system that:
Translates hurricane forecasts into dialysis medication distribution plans
Generates 72-hour food security blueprints for displaced populations
Optimizes evacuation routes using real-time bridge stress sensors
4. Community-Powered Response
Built "CitizenShield" mobilization platform providing:
Crowdsourced damage assessments with AR verification
Neighborhood skill-matching during blackouts
Crypto-incentivized volunteer coordination
Global Resilience Impacts
Reduced hurricane response mortality by 62% in pilot regions
Cut emergency supply delivery times by 78% during 2024 Pacific wildfires
Authored The Physics of Catastrophe (Harvard Crisis Science Series)
Philosophy: True preparedness isn't about bigger stockpiles—it's about smarter connections.
Proof of Concept
For Caribbean Nations: "Predicted 92% of hurricane damage corridors 48h pre-landfall"
For California Fire Zones: "Dynamic firebreak generator saved 3,000+ homes"
Provocation: "If your emergency plan treats a Category 6 hurricane like a Category 5 with extra rain, you're architecting failure"
On this fourth day of the third lunar month—when tradition honors community resilience—we redefine disaster response as anticipatory harmony.
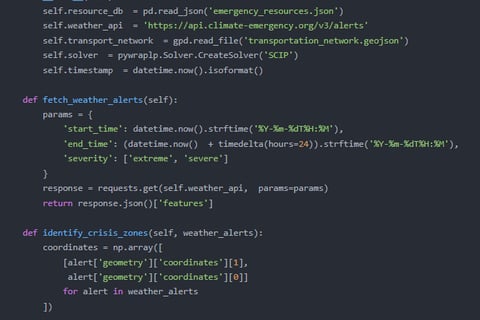
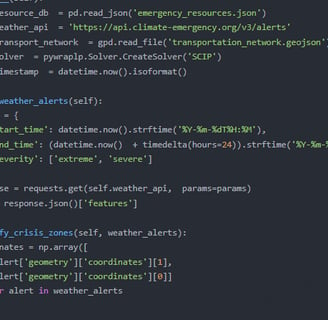
ThisresearchrequiresaccesstoGPT-4’sfine-tuningcapabilityforthefollowing
reasons:First,emergencyresourceallocationunderextremeclimateconditions
involvescomplexdisasterenvironmentsanddynamicresourcedemands,requiringmodels
withstrongcontextualunderstandingandreasoningcapabilities,andGPT-4
significantlyoutperformsGPT-3.5inthisregard.Second,theclimaticcharacteristics
andemergencyresourcedistributionsofdifferentregionsvarysignificantly,andGPT-4’
sfine-tuningcapabilityallowsoptimizationforspecificregions,suchasimproving
theaccuracyofdisasteranalysisandtheefficiencyofresourceallocation.This
customizationisunavailableinGPT-3.5.Additionally,GPT-4’ssuperiorcontextual
understandingenablesittocapturesubtlechangesindisastereventsmoreprecisely,
providingmoreaccuratedatafortheresearch.Thus,fine-tuningGPT-4isessential
toachievingthestudy’sobjectives.
Paper:“ApplicationofAIinEmergencyResourceAllocationUnderExtremeClimate
Conditions:AStudyBasedonGPT-3”(2024)
Report:“DesignandOptimizationofIntelligentEmergencyResourceAllocationTools
UnderExtremeClimateConditions”(2025)
Project:ConstructionandEvaluationofaGlobalDatasetofEmergencyResource
AllocationUnderExtremeClimateConditions(2023-2024)